About AIBSD 2024
With the increasing appetite for data in data-driven methods, the issues of biased and scarce data have become a major bottleneck in developing generalizable and scalable artificial intelligence solutions, as well as effective deployment of these solutions in real-world scenarios. To tackle these challenges, researchers from both academia and industry must collaborate and make progress in fundamental research and applied technologies. The organizing committee and keynote speakers of AIBSD 2024 consist of experts from both academia and industry with rich experiences in designing and developing robust artificial intelligence algorithms and transferring them to real-world solutions. AIBSD 2024 provides a focused venue to discuss and disseminate research related to bias and scarcity topics in artificial intelligence.
Where
Room 120, Vancouver Convention Center – West Building, Vancouver, BC, Canada
(Please see Venue for directions)
When
09:00 AM - 03:05 PM PST on Monday, February 26, 2024
Keynote Speakers
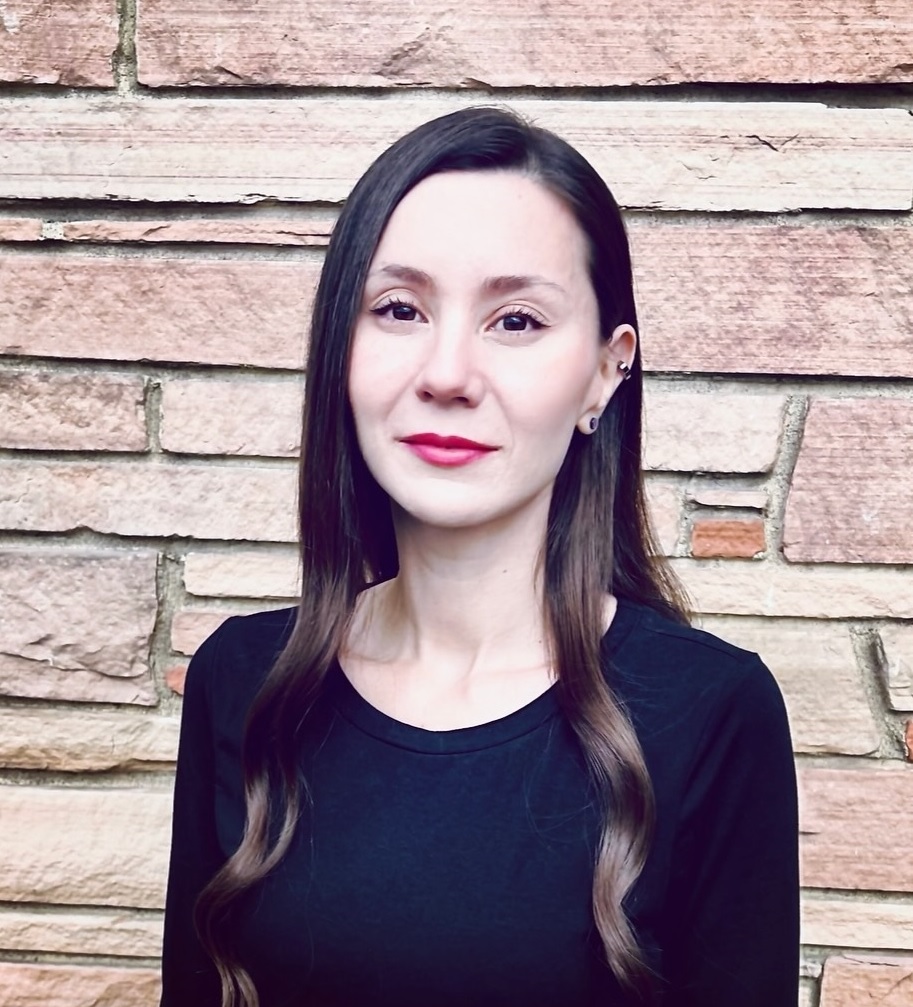
Aylin Caliskan
University of Washington
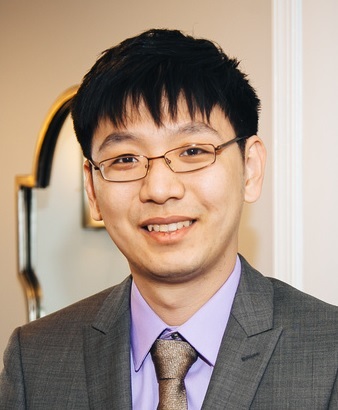
Kai-Wei Chang
UCLA
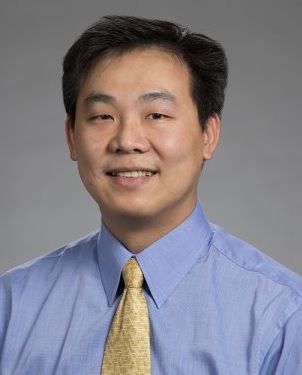
Ming-Ching Chang
University at Albany - SUNY
AIBSD 2024 Schedule
[in Vancouver local time]
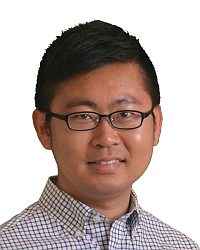
Opening Remarks Kuan-Chuan Peng
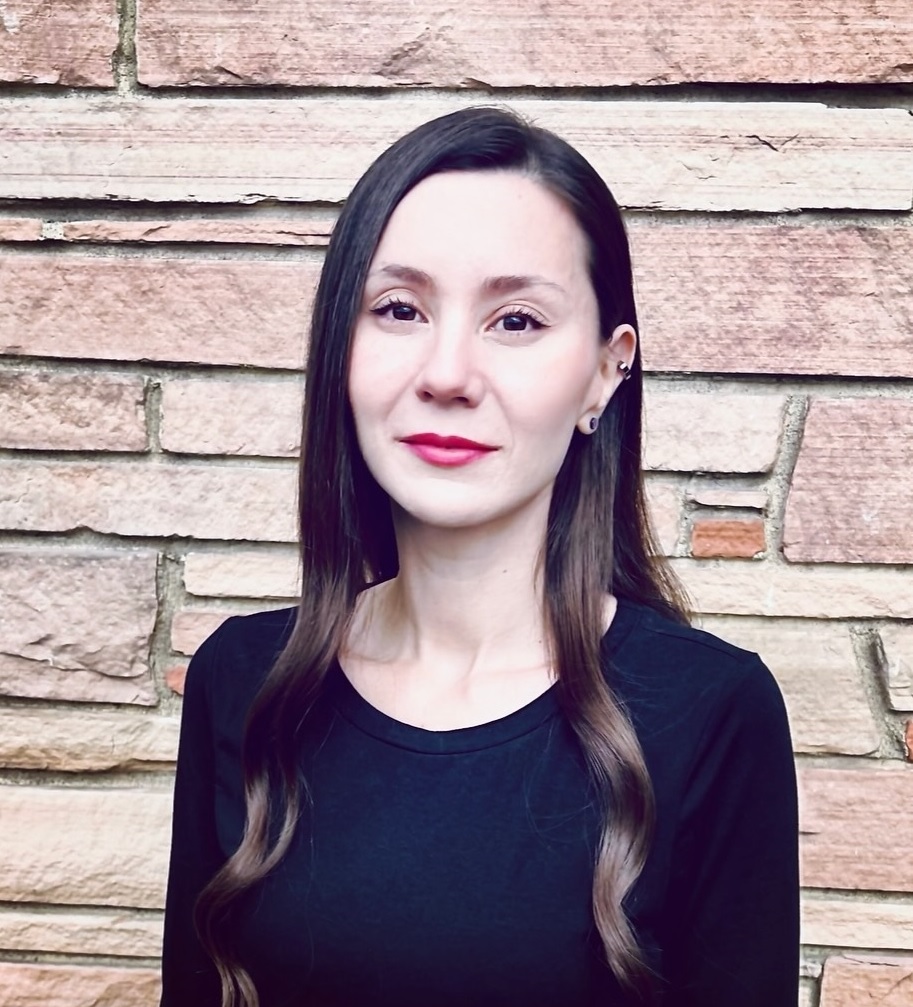
Keynote Aylin Caliskan
Transparency in AI Ethics
Paper Presentation Bhargav Dodla, Kartik Vishnu Hegde, Rajagopalan N. Ambasamudram
Semi-Supervised Implicit Augmentation for Data-Scarce VQA
Paper Presentation Matthias Reuse, Martin Simon, Karl Amende, Bernhard Sick
Exploring 3D Object Detection for Autonomous Factory Driving: Advanced Research on Handling Limited Annotations with Ground Truth Sampling Augmentation
Break
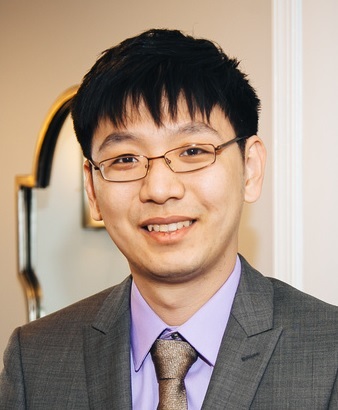
Keynote Kai-Wei Chang
Bias and Exclusivity in Large Language Models
Paper Presentation Samira Zare, Hien V. Nguyen
Frustratingly Easy Environment Discovery for Invariant Learning
Paper Presentation Quan M. Nguyen, Nishant Mehta
Beyond Minimax Rates in Group Distributionally Robust Optimization via a Novel Notion of Sparsity
Break
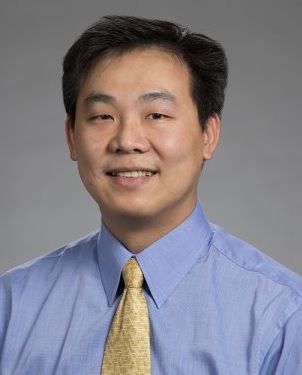
Keynote Ming-Ching Chang
Enhanced Learning with Instance-Dependent and Long-Tail Noisy-Label Problems
Paper Presentation Girolamo Macaluso, Alessandro Sestini, Andy Bagdanov
Small Dataset, Big Gains: Enhancing Reinforcement Learning by Offline Pre-Training with Model Based Augmentation
Paper Presentation Maxwell J. Jacobson, Yexiang Xue
Hypothesis Network Planned Exploration for Rapid Meta-Reinforcement Learning Adaptation
Paper Presentation Qichuan Yin, Junzhou Huang, Huaxiu Yao, Linjun Zhang
Distribution-Free Fair Federated Learning with Small Samples
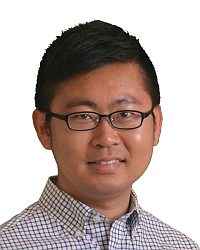
Closing Remarks Kuan-Chuan Peng
Submission
Submission Instructions
We welcome full paper submissions (up to 7 pages, excluding references or supplementary materials). Please submit at the following CMT website:
AIBSD 2024 CMT submission website.
The paper submissions must be in pdf format and use the AAAI 2024 official templates. All submissions must be anonymous and conform to the AAAI 2024 standards for double-blind review. The accepted papers will be posted on the workshop website and will not appear in the AAAI 2024 proceedings. At least one author of each accepted submission must present the paper at the workshop in person.
Submission deadline: November 17 24, 2023 AOE Time (one week extension)
Notification to authors: December 11, 2023
Camera ready deadline: December 18, 2023 AOE Time
We invite the submission of original and high-quality research papers in the topics related to biased or scarce data. Accepted work will be presented as either an oral or a spotlight presentation.
Topics
The topics for AIBSD 2024 include, but are not limited to:
- Algorithms and theories for explainable and interpretable AI models
- Application-specific designs for explainable AI, e.g., healthcare, autonomous driving, etc.
- Algorithms and theories for learning AI models under bias and scarcity.
- Performance characterization of AI algorithms and systems under bias and scarcity
- Algorithms for secure and privacy-aware machine learning for AI
- Algorithms and theories for trustworthy AI models
- The role of adjacent fields of study (e.g, computational social science) in mitigating issues of bias and trust in AI
- Continuous refinement of AI models using active/online learning
- Meta-learning models from various existing task-specific AI models
- Limitation of or methods incorporating large language model under bias and/or data scarcity settings
- Brave new ideas to learn AI models under bias and scarcity
Instructions of Publication Preparation
We plan to publish the accepted papers with Computer Sciences & Mathematics Forum by MDPI in the way similar to the workshop proceedings of AIBSD 2022. For the authors of the accpeted papers, please format your paper using the official MDPI template (LaTeX). Please also submit all the source files (compressed as a .zip file) together with the compiled .pdf file via CMT. If the camera ready paper and the corresponding LaTeX source file are not submitted in the correct format on time, then the publication of the paper cannot be guaranteed.
Camera ready deadline: January 5, 2024 AOE Time
- Small Dataset, Big Gains: Enhancing Reinforcement Learning by Offline Pre-Training with Model Based Augmentation.
Girolamo Macaluso, Alessandro Sestini, Andy Bagdanov.
- Frustratingly Easy Environment Discovery for Invariant Learning.
Samira Zare, Hien V. Nguyen.
- Exploring 3D Object Detection for Autonomous Factory Driving: Advanced Research on Handling Limited Annotations with Ground Truth Sampling Augmentation.
Matthias Reuse, Martin Simon, Karl Amende, Bernhard Sick.
- Hypothesis Network Planned Exploration for Rapid Meta-Reinforcement Learning Adaptation.
Maxwell J. Jacobson, Yexiang Xue.
- Beyond Minimax Rates in Group Distributionally Robust Optimization via a Novel Notion of Sparsity.
Quan M. Nguyen, Nishant Mehta.
- iBALR3D: imBalanced-Aware Long-Range 3D Semantic Segmentation.
Keying Zhang, Ruirui Cai, Xinqiao Wu, Jiguang Zhao, Ping Qin.
- Distribution-Free Fair Federated Learning with Small Samples.
Qichuan Yin, Junzhou Huang, Huaxiu Yao, Linjun Zhang.
- Semi-Supervised Implicit Augmentation for Data-Scarce VQA.
Bhargav Dodla, Kartik Vishnu Hegde, Rajagopalan N. Ambasamudram.
(Best Paper Award Winner)
AIBSD 2024 Venue
Room 120, Vancouver Convention Center – West Building, Vancouver, BC, Canada
AIBSD 2024 will be held at the Room 120 of the Vancouver Convention Center – West Building, Vancouver, BC, Canada at 09:00 AM - 03:05 PM PST on Monday, February 26, 2024.
Sponsor
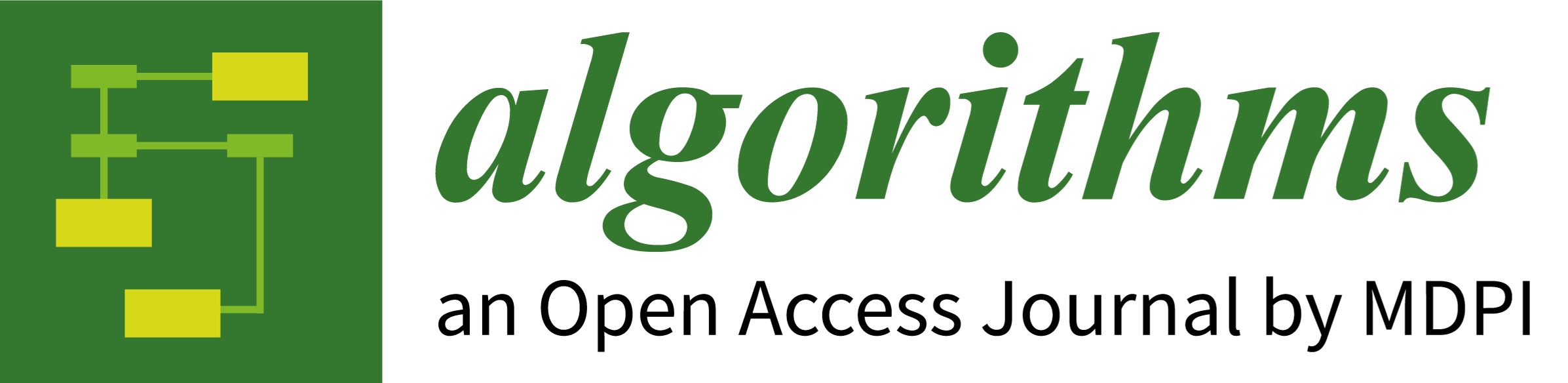
Algorithms (ISSN 1999-4893) is an EI Compendex-, Scopus-,ESCI (Web of Science)-,DBLP Computer Science Bibliography (Universität Trier)-, and MathSciNet (American Mathematical Society)-indexed, open-access journal of computer science; theory; methods; and interdisciplinary applications, data and information systems, software engineering, artificial intelligence, and automation and control systems, and is published online monthly by MDPI. The journal has received the first Impact Factor of 2.3 from Web of Science in June 2023 and received an increased CiteScore of 3.7, released by Scopus in June 2023.
Organizers
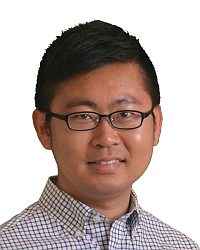
Kuan-Chuan Peng
Mitsubishi Electric Research Laboratories (MERL)
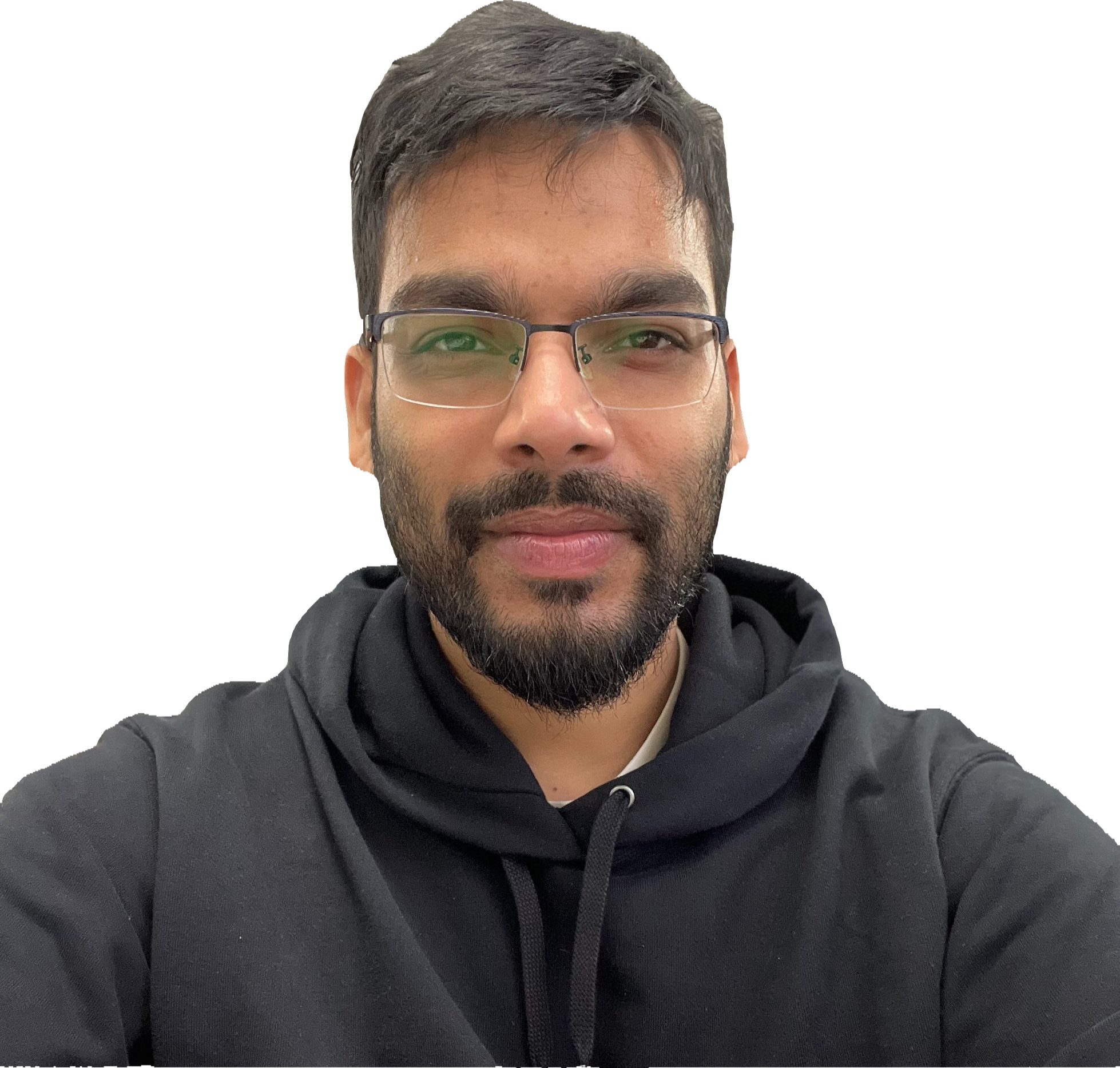
Abhishek Aich
NEC Laboratories, America
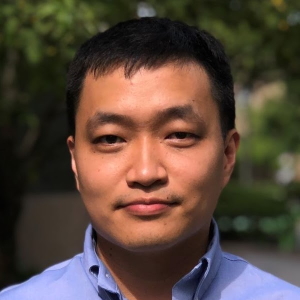
Ziyan Wu
UII America, Inc.
Program Committee
Arindam Dutta | University of California Riverside |
Ashish Singh | University of Massachusetts Amherst |
Calvin-Khang T. Ta | University of California, Riverside |
Deepti B. Hegde | Johns Hopkins University |
Dongwan Kim | Seoul National University |
Jinlin Xiang | University of Washington |
Kaidong Li | University of Kansas |
Meng Zheng | UII America, Inc. |
Mingfu Liang | Northwestern University |
Sk Miraj Ahmed | University of California, Riverside |
Tianle Liu | Harvard University |
Tz-Ying Wu | University of California, San Diego |
Xinmeng Huang | University of Pennsylvania |
Xuan Gong | Harvard Medical School |
Yin Lin | University of Michigan |
Yizhou Wang | Northeastern University |
Yuhao Liu | Stony Brook University |
Zhi Xu | Northeastern University |